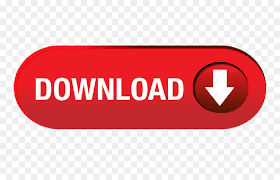

Set the alarm to trigger when the posterior probability of a fire exceeds 0.02 while at home and at 0.01 while away Type I error for smoke detector: probability of alarm given no fire=0.05īayesian: probability of fire given current air characteristicsįrequentist smoke alarm designed as most research is done: Set the alarm trigger so as to have a 0.8 chance of detecting an infernoĪdvantage of actionable evidence quantification: Subjective frequentist interpretation of a rather meaningless probability is not and thus not subject to informed challenge. Null hypothesis testing is simple because it kicks down the road the gymnastics needed to subjectively convert observations about data to evidence about parameters. The subjectivist (i.e. Bayesian) states his judgements, whereas the objectivist sweeps them under the carpet by calling assumptions knowledge, and he basks in the glorious objectivity of science. When was the last time you observed a sampling distribution, empirically and objectively? When was the last time you saw someone justify their choice of a sampling distribution, or tested it? – Stephen Martin

Those who criticize Bayes for having to choose a prior must remember that the frequentist approach leads to different p-values on the same data depending on how intentions are handled (e.g., observing 6 heads out of 10 tosses vs. having to toss 10 times to observe 6 heads accounting for earlier inconsequential data looks in sequential testing). The frequentist approach stays close to home, not requiring quantification of prior knowledge, to answer an easier but almost irrelevant question (how strange are my data)Īny frequentist criticizing the Bayesian paradigm for requiring one to choose a prior distribution must recognize that she has a possibly more daunting task: to completely specify the experimental design, sampling scheme, and data generating process that were actually used and would be infinitely replicated to allow p-values and confidence limits to be computed. The Bayesian approach goes out on a limb in order to answer the original question (is an effect > x). Wrong question: How surprising is our result if the treatment has no effect at all? Right question: Does the treament work more than a trivial amount?Įxact answer: Under the simplifying assumption that H 0 is true we can actually compute exact p-values in 1/ 10 of the models we use Bayes is about the θ generating process, and about the data generate d.įar better an approximate answer to the right question, which is often vague, than the exact answer to the wrong question, which can always be made precise – John TukeyĪpproximate: My prior distribution disagrees with yours, so my posterior probability that the treatment works is 0.02 less than yours (and BTW the p-value from the frequentist analysis is only accurate to ☐.015 in this non-normal case) With frequentism, you make assumptions about the process that generated your data and infinitely many replications of them, and try to build evidence for what θ is not.įrequentism is about the data generating process. With Bayes you start with a prior distribution for θ and given your data make an inference about the θ-driven process generating your data (whatever that process happened to be), to quantify evidence for every possible value of θ. The difference between Bayesian and frequentist inference in a nutshell:
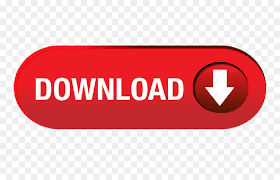